Global weather models form the foundation of many of the available solar irradiance and power forecasting models, and are typically sourced from publicly funded Weather Agencies, including the European Centre for Medium-Range Weather Forecasts (ECMWF).
In late February, the ECMWF added cloud and radiation outputs to their first operational AI-driven weather model, AIFS V1, offering a new kind of solar radiation forecasting. The AIFS V1 operational release is a pivotal moment for solar AI and energy forecasting as it is the first operational "AI model" to contain cloud cover and solar radiation parameters.
At Solcast, our team was eager to assess how well this new AI-driven solar model performs when it comes to forecasting solar irradiance and whether it may add value to our current estimates. Our Data Scientists conducted an early analysis, benchmarking AIFS forecasts against Solcast’s globally validated actuals. Despite being in its early stages, AIFS already shows competitive performance, with promising accuracy metrics across multiple forecast horizons.
What is AIFS and why does it matter for solar forecasting?
Accurate, large scale solar radiation forecasts are crucial for grid firming, helping to balance supply and demand as solar power increases as a share of energy generation. We have recently seen the release of multiple AI Numerical Weather Prediction models, but until recently they all focused on temperature, wind, and precipitation, leaving solar irradiance parameters unexplored. The model released by the ECMWF is the first to explicitly model cloud cover and solar radiation. These models are very interesting because they are much cheaper and faster to run, as well as being much easier to handle and understand. At the core of AIFS is a Graph Neural Network, an architecture popularized in weather by Deepmind’s GraphCast 2023 Science paper, that suits very well the spatial nature of weather.
AIFS is still running at a lower spatial and temporal resolution than its physical cousin, the IFS, the current state of the art global NWP by the ECMWF with 6 hours timesteps and 0.25deg resolution, as you can see in the comparison below. However the results are very promising!
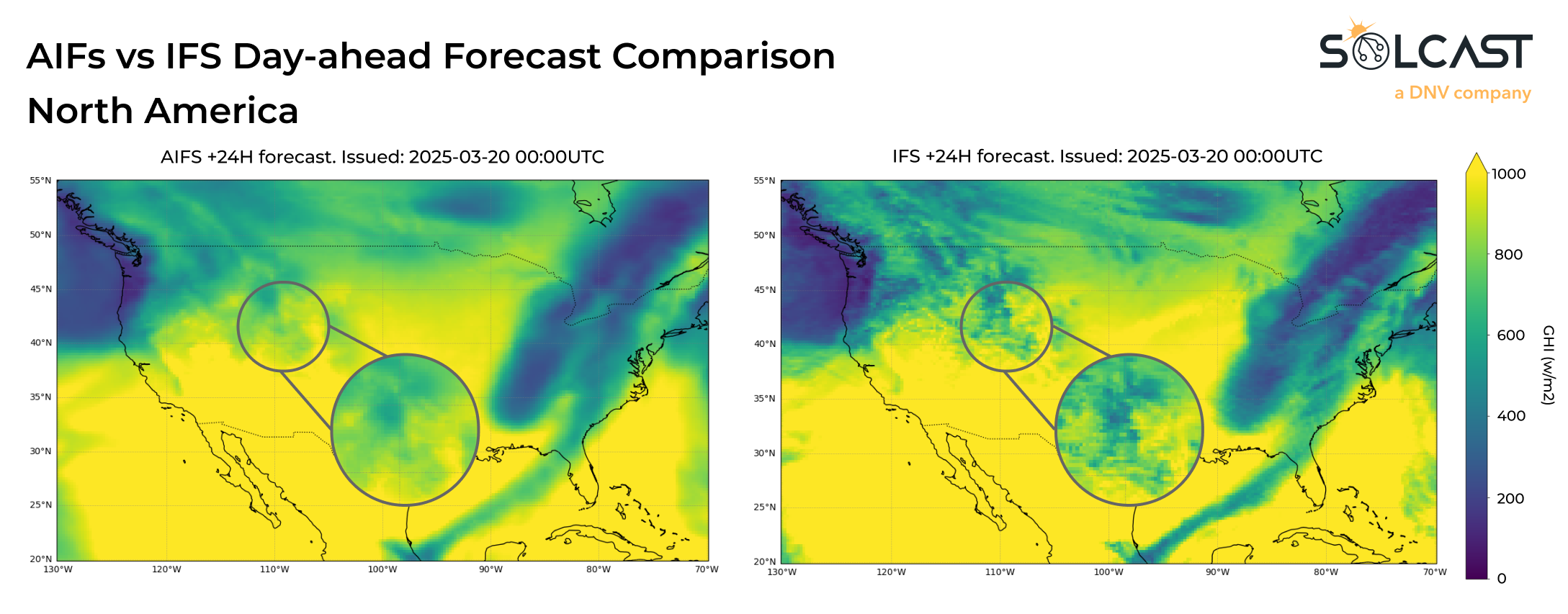
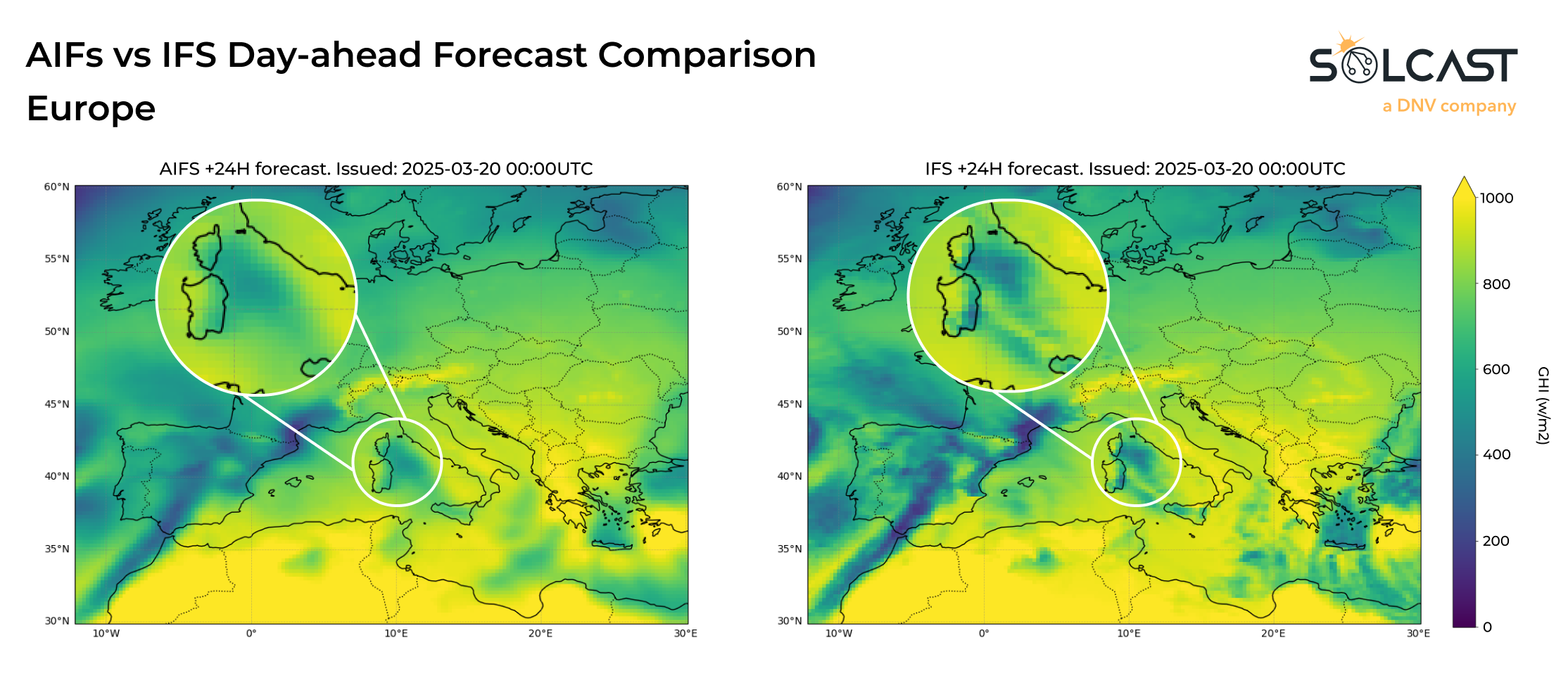
We can expect future releases of the AIFS to be at higher temporal and spatial resolution as well as increased update frequency and it will be fascinating to see how the industry can take advantage of this model’s faster (and cheaper) run time to deliver more accurate probabilistic and ensemble forecasts.
How does the AIFS model forecast perform against traditional models?
Comparison analysis methodology
To better understand AIFS performance in real-world solar forecasting context, we ran a comparative analysis using Solcast actuals as the benchmark. Our data science team compared the forecast performance of AIFS against two well-regarded global weather models (IFS and GFS) over the weeks since AIFS was published. While we acknowledge that the dataset is still limited, these initial results provide a preliminary look at performance trends.
We benchmarked the models against Solcast actuals, which offer the best estimate of real-world solar irradiance conditions. Solcast actuals are derived using satellite imagery, advanced modeling algorithms, and validated globally to have significantly lower bias and Mean Absolute Error (MAE) than reanalysis datasets like ERA5, according to the 2022 global accuracy analysis.
For this assessment, we analysed day-ahead forecasts at +1, +3, +5 days for most capitals worldwide. The model performance was then evaluated using two key error metrics: normalised Mean Absolute Error (nMAE) and normalised bias. Together, these metrics provide insights into both forecast accuracy and bias tendencies which are critical for real-world applications.
Findings from global solar forecast analysis of AIFS, IFS, GFS models
ECMWF’s own early evaluation highlights a tendency for AIFS to favor intermediate cloud conditions, under-representing in both clear-sky and heavily overcast scenarios. This results in solar radiation forecasts that are less active than those from IFS, leading to a more conservative estimation of irradiance.

The Solcast team found the same conclusion, with good Mean Average Error (nMAE), but a negative bias. AIFS consistently under-forecasts irradiance across all horizons, with a bias of approximately -8%. In contrast, IFS shows a slight positive bias of +2%, and GFS leans higher at +5%.

Despite these biases, AIFS V1 performs well in capturing variance. Analyzing normalized Mean Absolute Error (nMAE) values, the model matches IFS across all forecast horizons and outperforms GFS by a significant margin. This level of performance is impressive, especially considering that AIFS is still only running at 25km resolution.

How AI-based weather models improve solar forecasting accuracy
AIFS V1 remains in its early stages, with further refinements needed. Nonetheless, it represents a significant step forward in AI-based weather modelling and could complement existing numerical approaches. ECMWF’s efforts to operationalize AI-driven forecasting is an exciting evolution in meteorology, with potential long-term benefits for solar energy forecasting and grid stability.
As AI solar and weather models continue to evolve, the Solcast team is looking at how we can integrate these advancements into our models to help drive better forecasting outcomes for the solar industry.
If you want to learn more about how we’re using AI to further enhance our forecasts, reach out to our team. In the meantime, you can check our live and forecast accuracy, or see how our forecasts performed in an independent forecast accuracy trial.
